AI's Growing Demand Signals the Short-Lived Boom of the Chip Industry
The recent growth in the AI industry has brought new attention to the chip sector. In this article, we will look deeper into this interesting topic. We’ll discuss AI’s demand for chips, the limits of Moore’s Law, and whether AI itself could be the force that ends the chip industry’s growth.
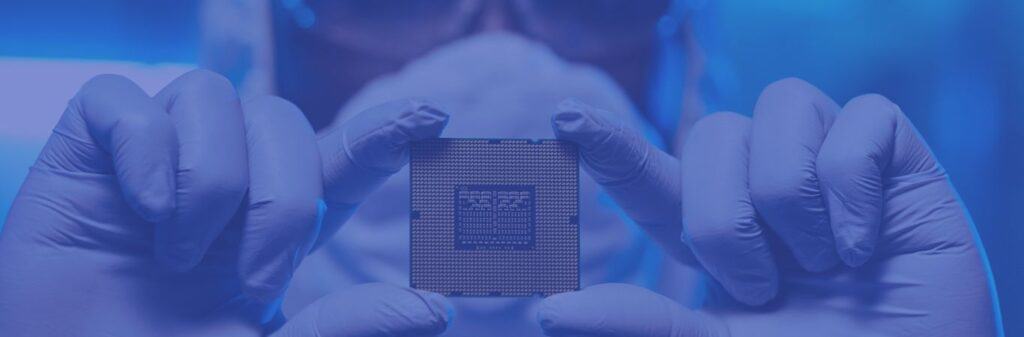
Computing Power Reaches a Bottleneck
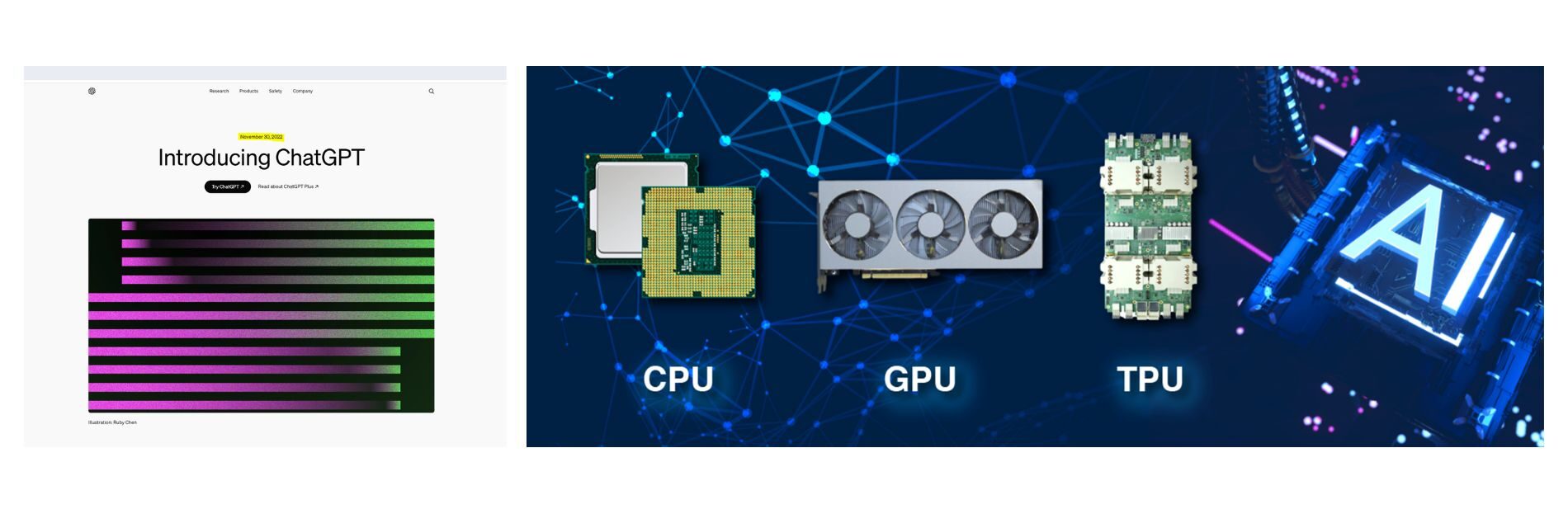
First, let’s review the development of artificial intelligence. The year 2022 was named the “Year of AI.” This marked the official start of the AI era.
AI’s progress has relied on improvements in computing power. It has moved from using traditional CPUs to advanced GPUs, TPUs, and NPUs. Each of these developments has boosted the semiconductor industry’s growth.
Due to AI’s rapid evolution, companies like Nvidia have seen sharp rises in their stock prices. Nvidia is now the second-largest corporation by market value.
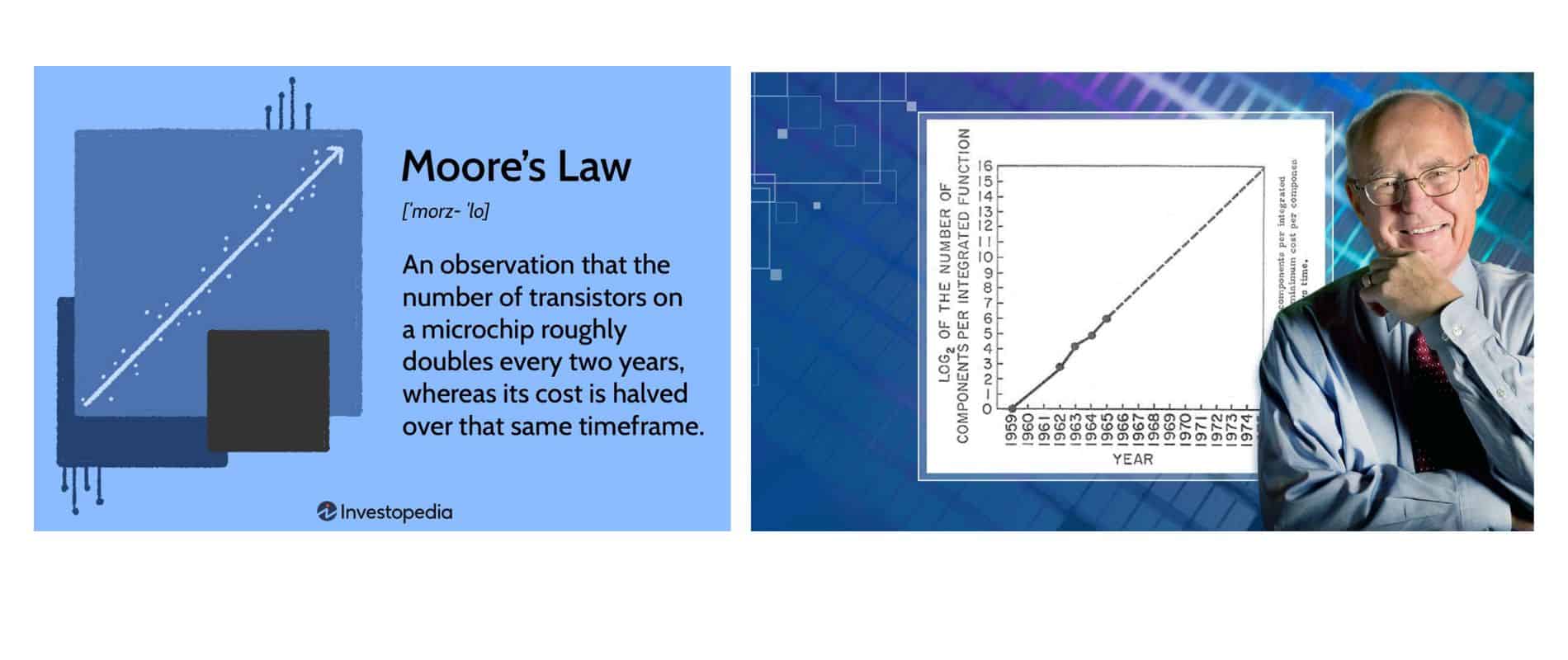
As technology advances, the limits of Moore’s Law are becoming clearer. Since the late 20th century, Moore’s Law has driven technological progress and changed society.
Many companies, like Facebook, Amazon, Google, and Apple (known as FAGA), based their business models on Moore’s insights. However, as transistor counts rise, power consumption also increases, reducing efficiency.
CEOs like Jensen Huang have stated for years that Moore’s Law is outdated. In a 2005 interview, Moore himself acknowledged the physical limits of his prediction.
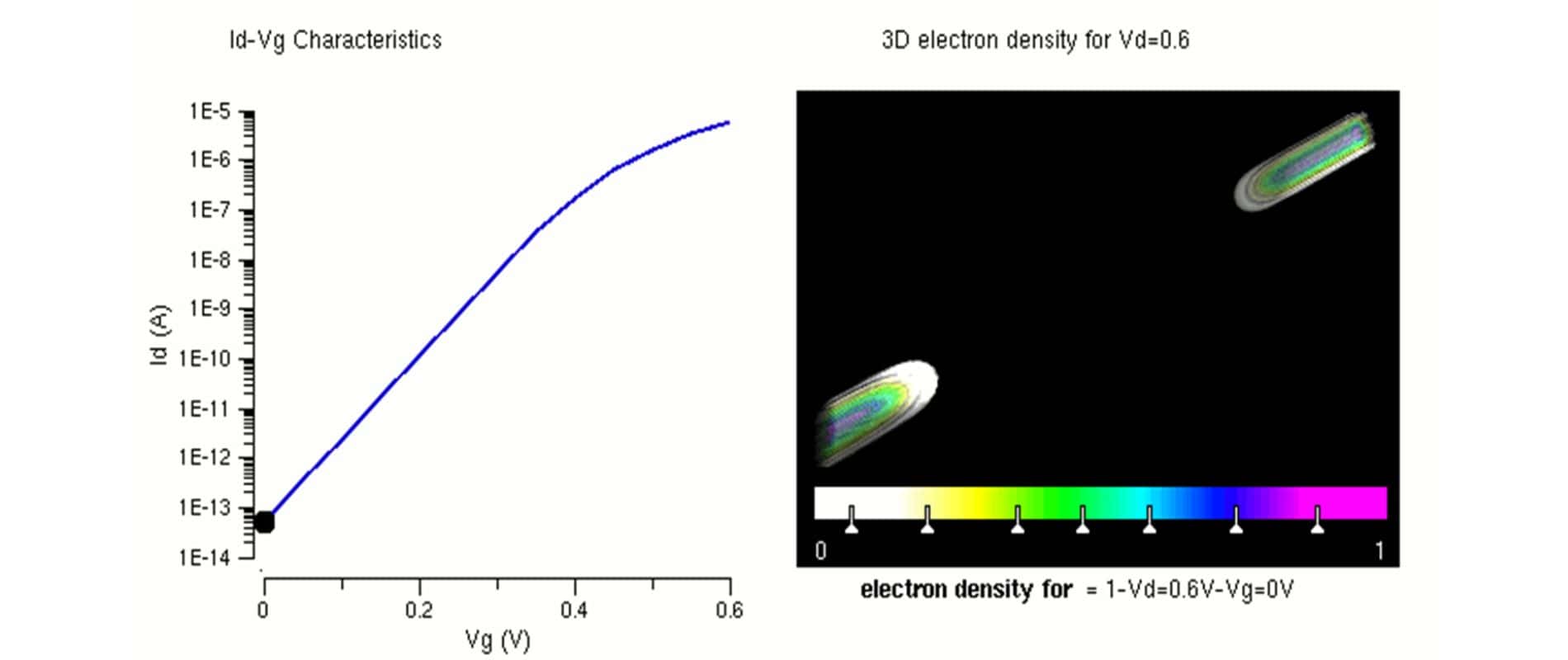
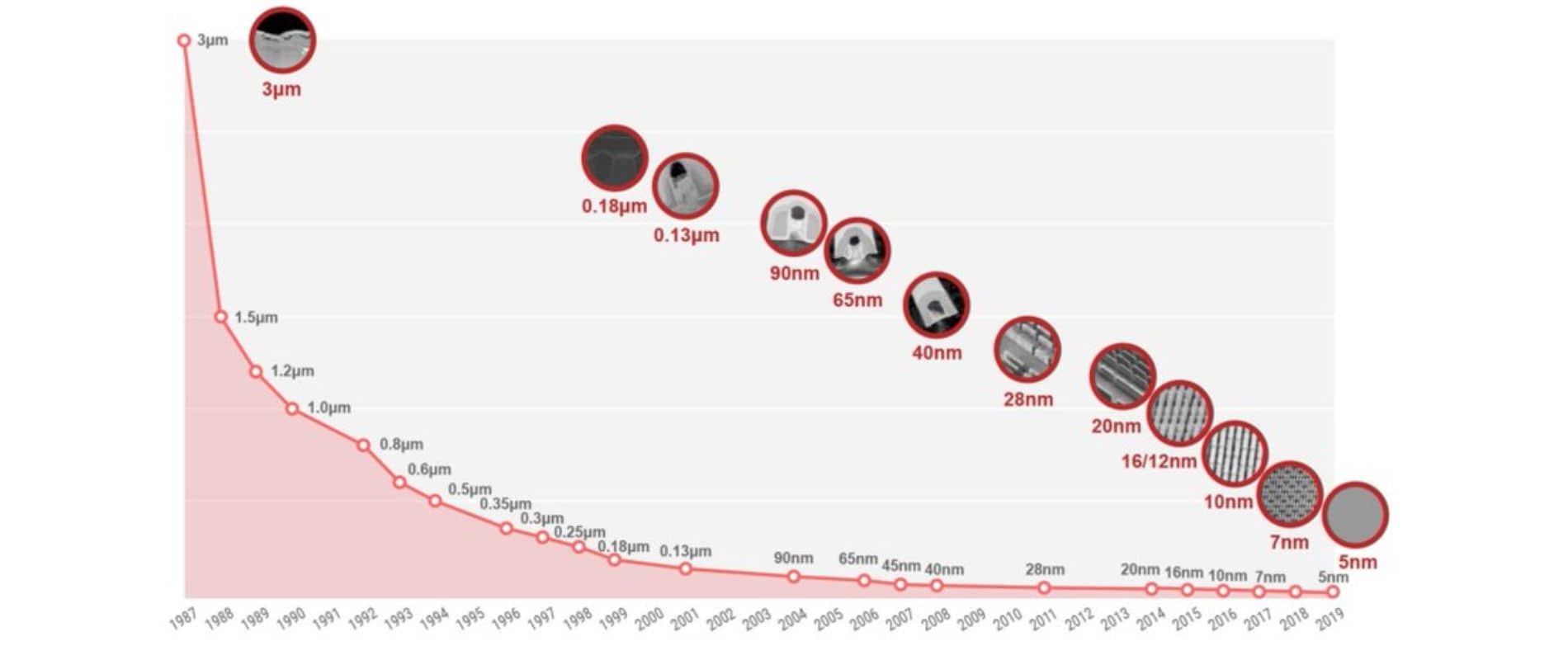
Can Moore’s Law really keep being upgraded indefinitely?
Historically, advances in process technology have driven Moore’s Law forward. In the shift from 3µm to 0.13µm, Dennard Scaling worked well. But after 0.13µm, Dennard Scaling began to fail. The main issue is that as transistors get smaller and their numbers increase, constant chip sizes cause current leakage in the channel area. This leakage heats up transistors, increasing power use and breaking the simple link between transistor count, speed, and energy use.
Today, CPU manufacturers can’t rely on simply raising clock speeds and core counts to boost power. Rising power consumption means only some cores can run at optimal performance.
The industry has tried various ways to extend Moore’s Law, researching and experimenting extensively. But these efforts haven’t fixed the central issue: heat buildup, which ultimately reduces performance.
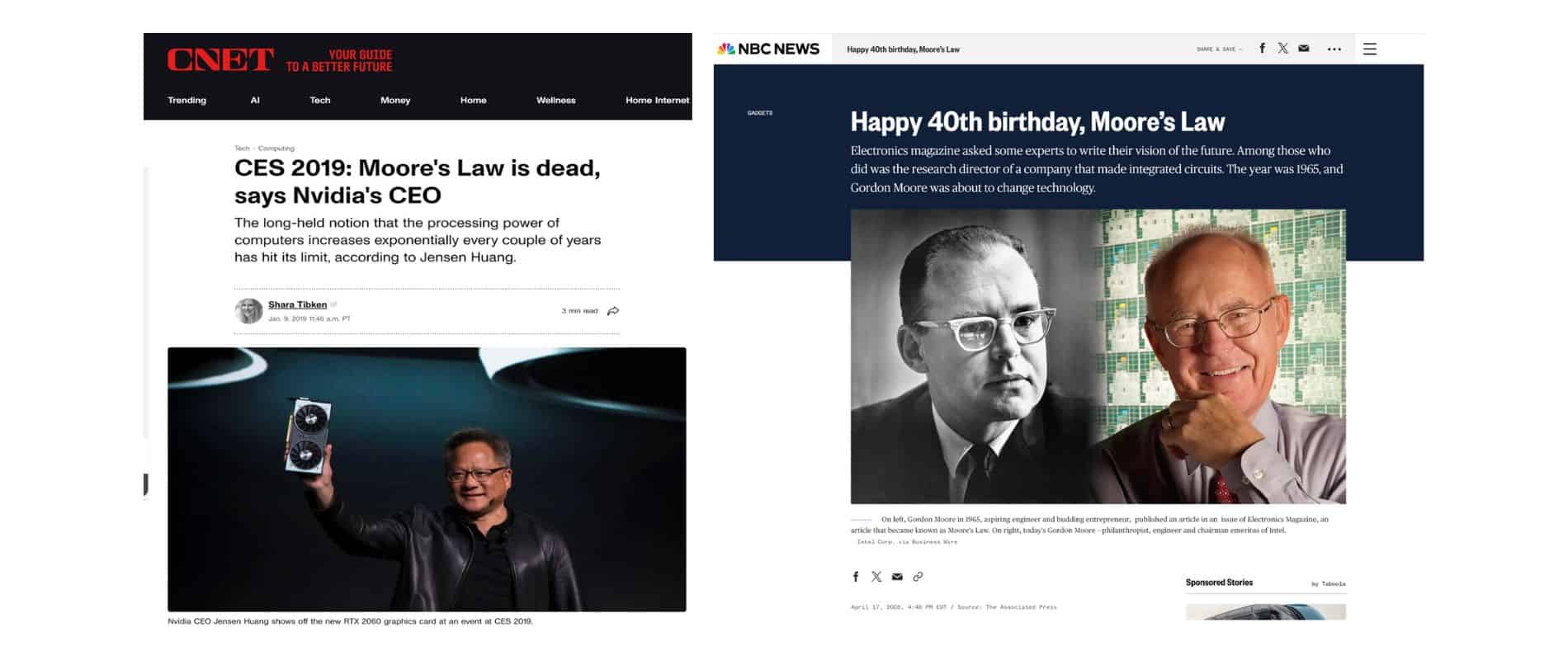
In 2023, the passing of Moore’s Law creator, Gordon Moore, marked the end of an era in the chip industry. As technology advances, we may need to shift away from a reliance on chips and explore new technological paths. The physical limitations of chips have created a bottleneck in technological progress, and breaking through this barrier may require moving beyond the chip industry. Thus, the chip industry could be considered a sunset industry.
Beyond technological constraints, industry collaborations and innovations are noteworthy. For instance, OpenAI CEO Sam Altman recently took a nuclear energy company public to support AI advancements. These cross-industry collaborations could represent a future direction for technological growth.
When considering AI’s impact on the chip industry, the demand for data centers is crucial. Training AI models requires extensive data processing and storage, driving the need for high-performance chips and high-speed networks. Cloud giants like Google, Amazon, and Microsoft have heavily invested in new data center architectures to meet these needs. For example, Google’s TPU chips have become essential in the cloud computing market, outperforming traditional CPUs and GPUs in machine learning tasks.
Additionally, AI is fueling the rise of edge computing. Edge computing brings data processing closer to its source, reducing latency and boosting efficiency. This shift has spurred demand for low-power, high-performance chips that can run complex AI algorithms on edge devices. To address this market, chip manufacturers and tech companies are now developing new architectures tailored to edge computing.
Another trend is the diversification of AI hardware. While GPUs were once the standard for training deep learning models, the growth of AI has led to custom chips designed for specific tasks. For instance, Google’s TPU accelerates neural network inference, while Intel’s Nervana chip focuses on deep learning training tasks. These specialized hardware designs enhance AI application performance and drive further innovation in the chip industry.
In summary, AI’s rapid development is significantly shaping the chip industry. As technology evolves, AI will continue to push demands on chip design, manufacturing, and applications. This creates both new opportunities and challenges, underscoring the need for continued innovation.
AI's power demand is virtually limitless
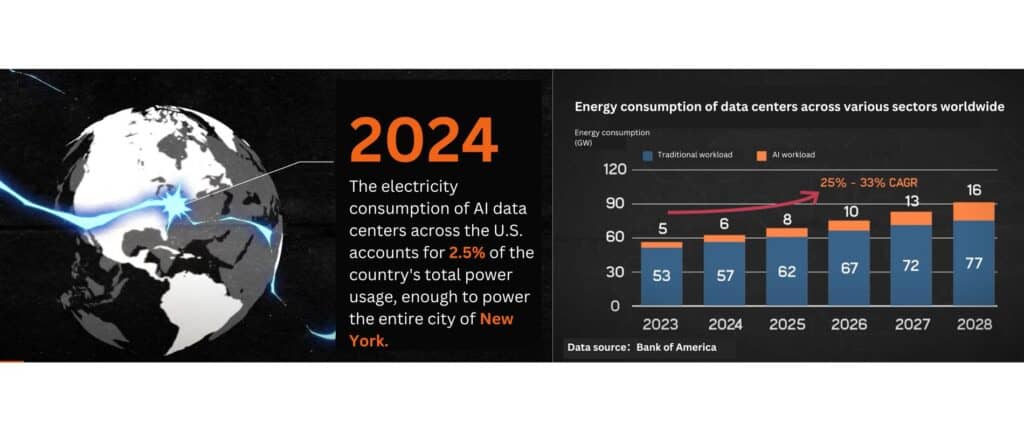
AI’s growing demand for chips is ironically driving it to become the very force that could end the chip industry. At the core of this trend is AI’s enormous power consumption. This turns AI into an energy-devouring beast.
For instance, training an AI model like ChatGPT consumes around 1,300 megawatt-hours of electricity daily. To put this in perspective, that’s enough energy to stream online videos for 185 years. This highlights the staggering power requirements of AI.
As AI advances rapidly, power consumption in AI data centers across the U.S. now accounts for 2.5% of the nation’s total electricity usage. This is a staggering amount—enough to power the entire city of New York.
From 2020 to 2022, global data center electricity consumption surged from 200-250 TWh to 460 TWh. This now represents 2% of global electricity usage.
What’s more concerning is the growth rate. This figure is rising annually by 25% to 33%. Projections suggest it will reach 1,000 TWh by 2026, which is equivalent to Japan’s total electricity usage.
In addition to the power used by IT equipment, cooling systems also demand significant energy to maintain optimal temperatures. For every unit of energy consumed by IT equipment, cooling systems require an additional 0.4 units.
Altogether, data centers consume 1.4 times the energy needed solely for IT operations. This underscores the immense power required, not just for computing, but also for keeping these systems running.
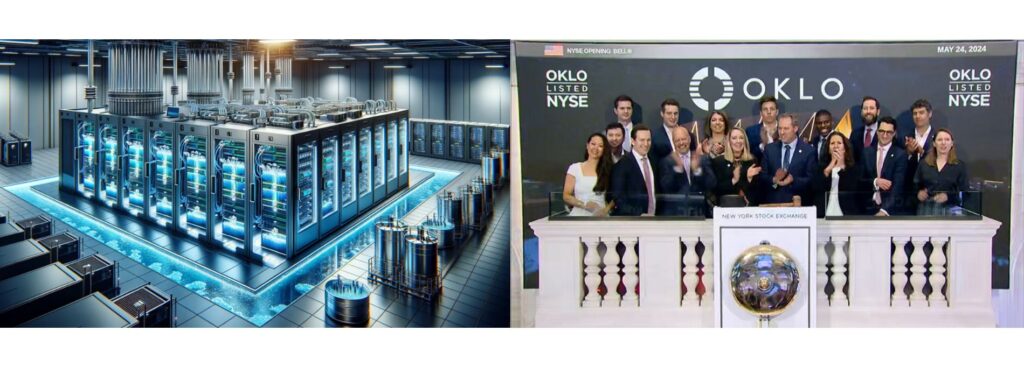
As a result, companies worldwide are fiercely competing for electricity, creating what can be called a “power race.” This race for energy is one reason OpenAI helped nuclear power company Oklo go public.
Traditional power sources can no longer meet AI’s colossal energy demands, making nuclear power a potential solution. However, building nuclear plants takes time, and a major factor limiting AI’s growth is the shortage of electricity.
By supporting the listing of a nuclear power company, OpenAI aims to accelerate the expansion of energy infrastructure to keep up with AI’s ever-growing energy needs.
What Should the 'Chip Terminator' Look Like?
Let’s explore the characteristics and features that the ‘Chip Terminator’ should possess.
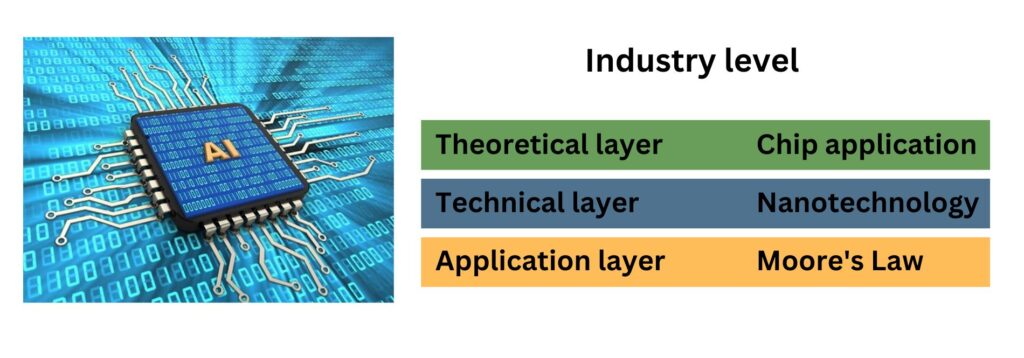
As AI’s demand for chips—and computing power—continues to rise, existing chips are no longer enough to support AI’s rapid growth. In fact, they have become a barrier to further advancements.
In the past, Moore’s Law drove constant improvements in chip performance. But over time, maintaining this law has become harder, with nanotechnology reaching its limits. This bottleneck means modern chips can no longer advance at the pace Moore’s Law once promised, signaling the start of their obsolescence.
To solve this, new technologies must replace current chips. We need to look at three layers in this process: theoretical, technological, and application. Moore’s Law forms the theoretical layer, offering foundational principles for chip growth. Nanotechnology, the technological layer, has allowed Moore’s Law to continue through manufacturing improvements. But as Moore’s Law becomes unsustainable and nanotechnology nears its limits, chip applications are also reaching an endpoint.
The chip industry is now entering a twilight phase, moving through the natural stages of industry development: first theory, then technology, and finally application.
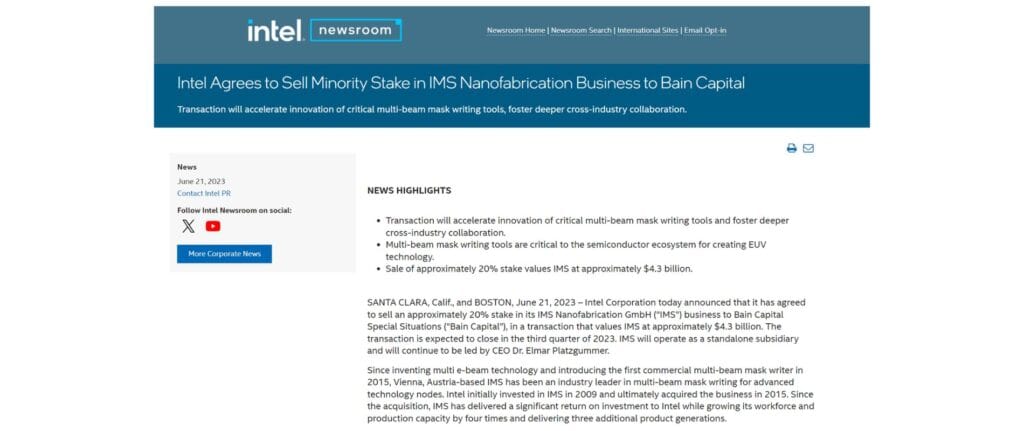
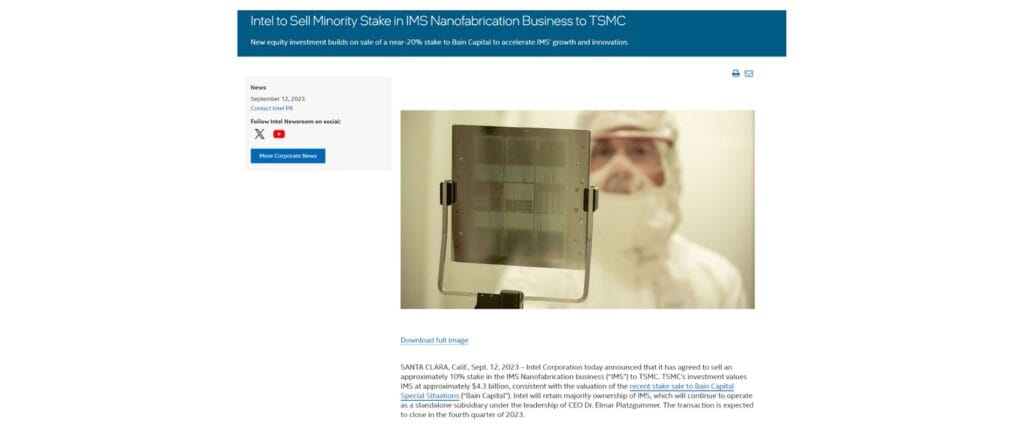
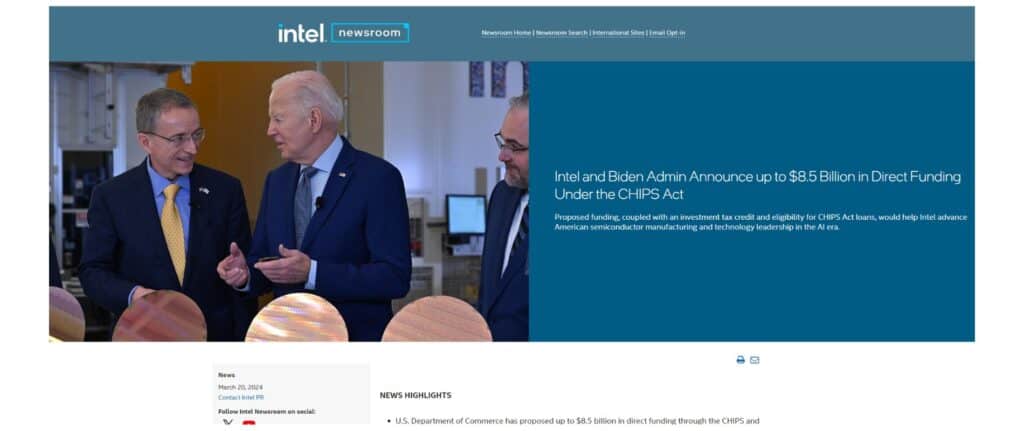
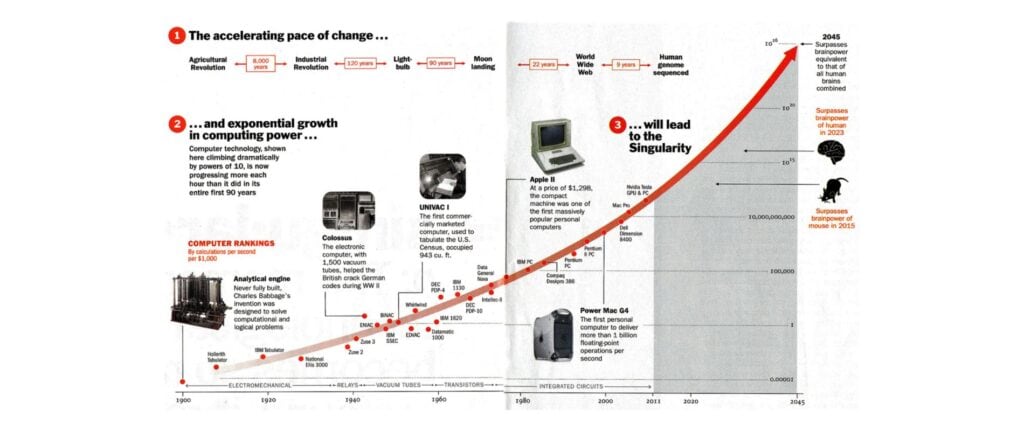
For this reason, we shouldn’t be swayed by superficial trends. Today’s leading chip manufacturers, like Nvidia, may only be leveraging existing technology before it’s phased out. Consider Intel, a key player in the semiconductor field. Recently, Intel has sold off parts of its nanotechnology and patents—not due to a lack of skill, but because it recognizes that semiconductor technology is nearing a bottleneck and must shift toward new fields.
During this transition period, addressing power consumption and heat dissipation is essential. Current chips produce too much heat during high-performance computing, requiring significant power for cooling. This not only wastes resources but also restricts further improvements in chip performance.
Thus, the next generation of chip technology—dubbed the “chip terminator”—must tackle these issues. By incorporating new materials, structures, or techniques, the aim is to lower power usage and improve heat dissipation efficiency.
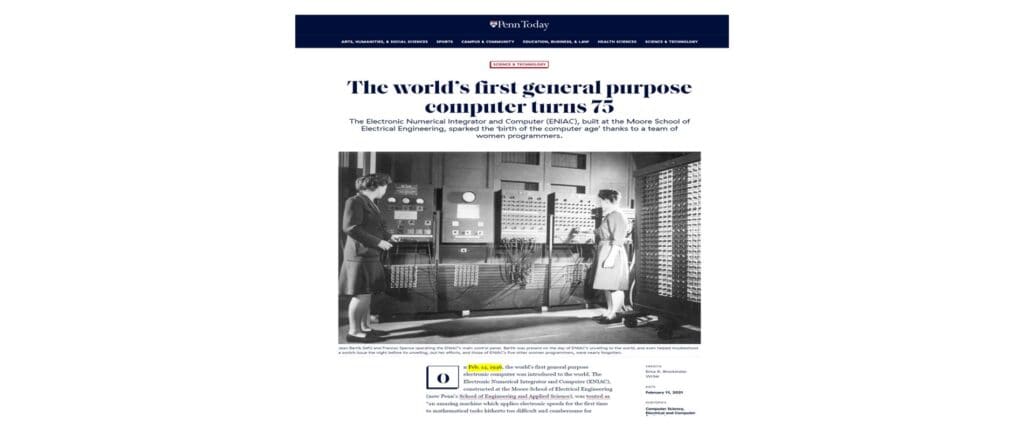
While the true “chip terminator” is still on the horizon, the path of technological progress is becoming clear. Similar to the evolution of computers, AI will transition from being bulky and power-hungry to smaller and more efficient. This shift may even occur faster than the computer industry’s transformation.
Therefore, closely following advancements in technology is essential to staying ready for the next wave of innovation.
How to Invest?
With the Fourth Industrial Revolution, driven by AI, big data, IoT, and cloud computing, we stand on the brink of a rapid leap in technological progress. This shift will inevitably generate immense wealth. As everything accelerates, choosing the right investment direction is critical. A wrong choice could mean not just missed profits, but also substantial losses.
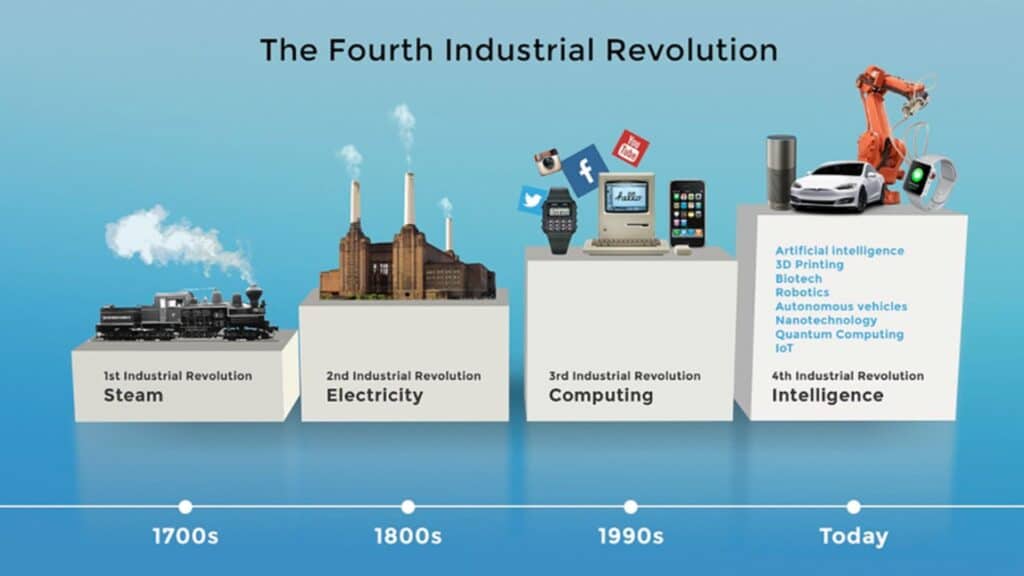
At Ai Financial, our strategy includes a “do not do” list. The fifth item is “do not invest in sunset industries.” So, as discussed in this lecture, is the chip industry a sunrise or sunset industry?
From today’s session, it’s clear: artificial intelligence (AI) is a sunrise industry, while chips belong to a sunset industry. Therefore, in making investment decisions, we should steer clear of sunset industries, including the chip sector. Consequently, Nvidia stock may not be a suitable purchase.
Investing in stocks is essentially about probabilities. Speculation often leads to losses rather than profits. The better approach is to invest in public principal-protected funds. These funds hold a diversified portfolio, ensuring that even if one stock underperforms, others may perform well, reducing risk. For instance, over the past two years, our funds have captured Nvidia’s (NVDR) rise, highlighting the benefits of public funds.
The optimal strategy, then, is to use investment loans to buy public principal-protected funds. This approach allows us to capture stock market gains while maintaining a balanced asset allocation.
You may also interested in
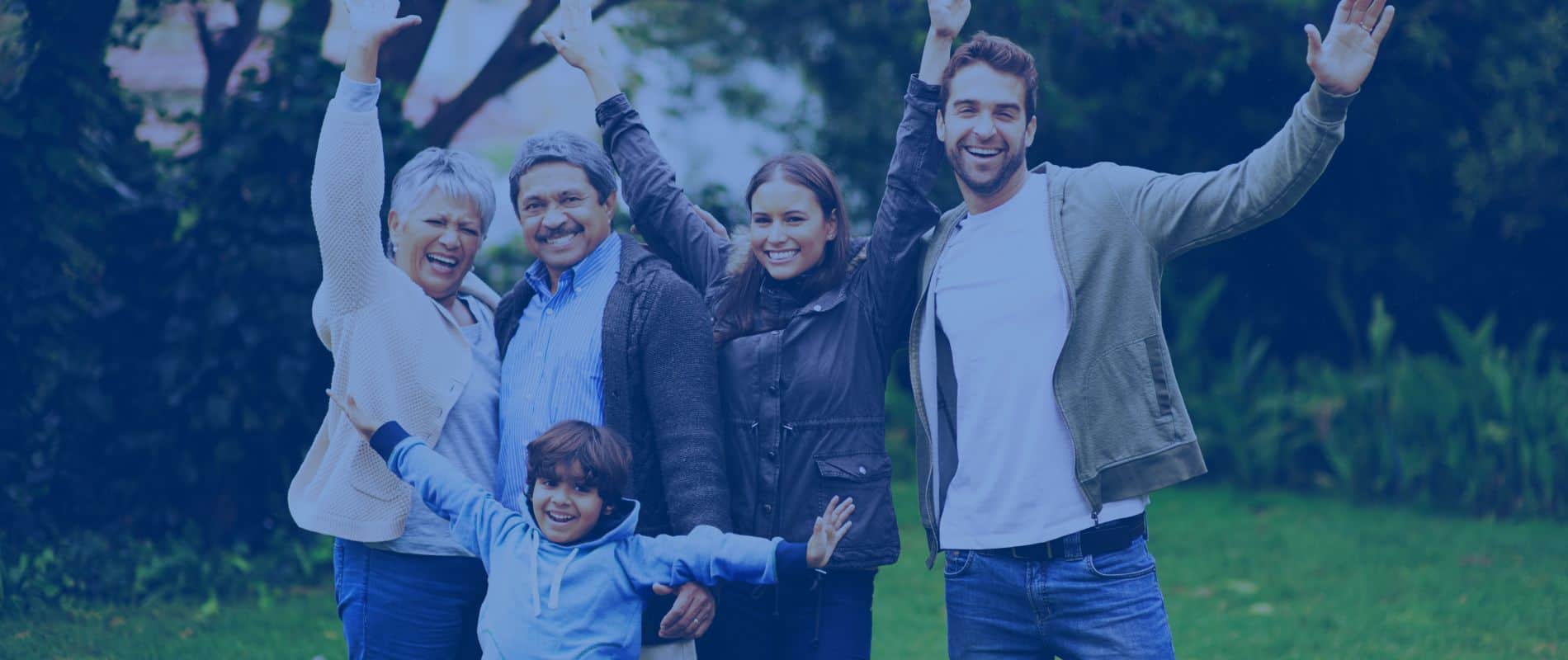
What is an investment loan?
Can this loan last a lifetime? Interest-only payments? Tax-deductible? Is it a private loan? Is the threshold high?
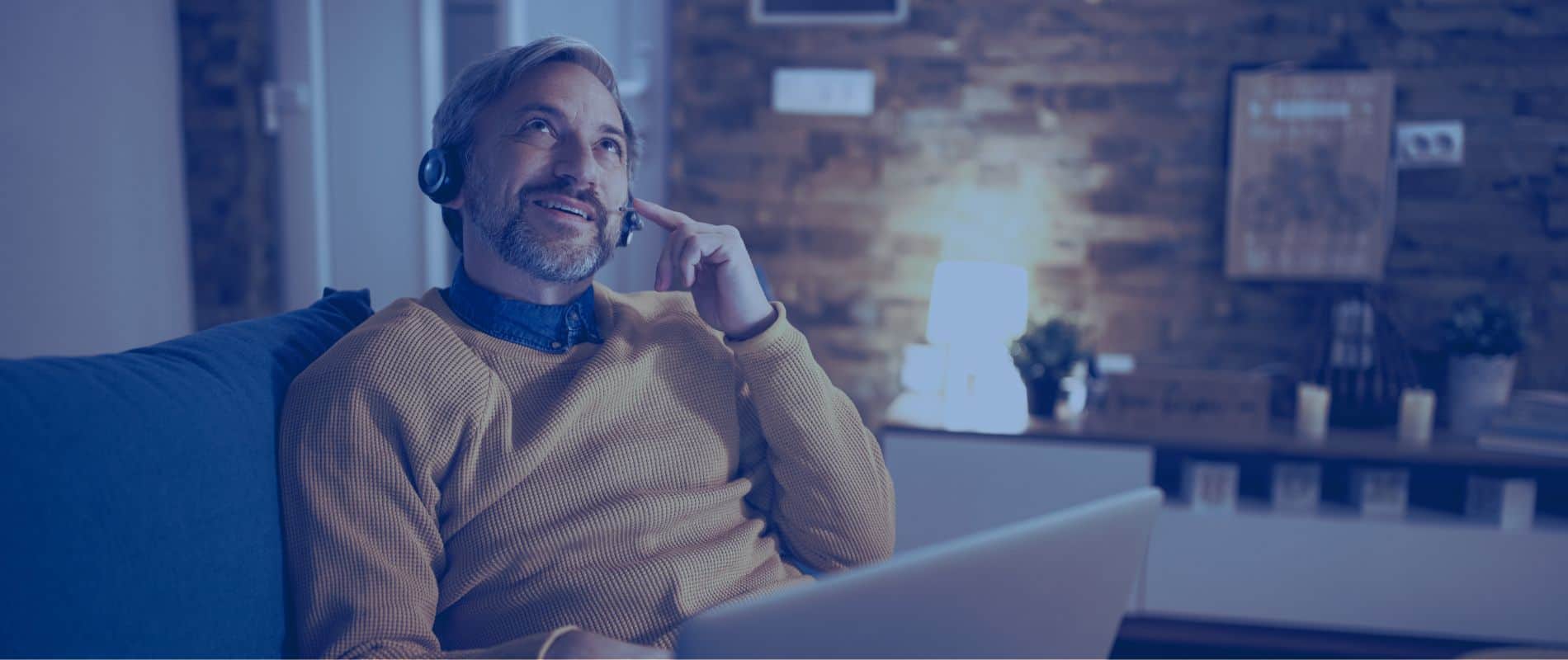
Why do you need segregated funds for retirement?
Segregated funds are a popular choice for group savings and retirement plans. They provide access to high-end and unique……
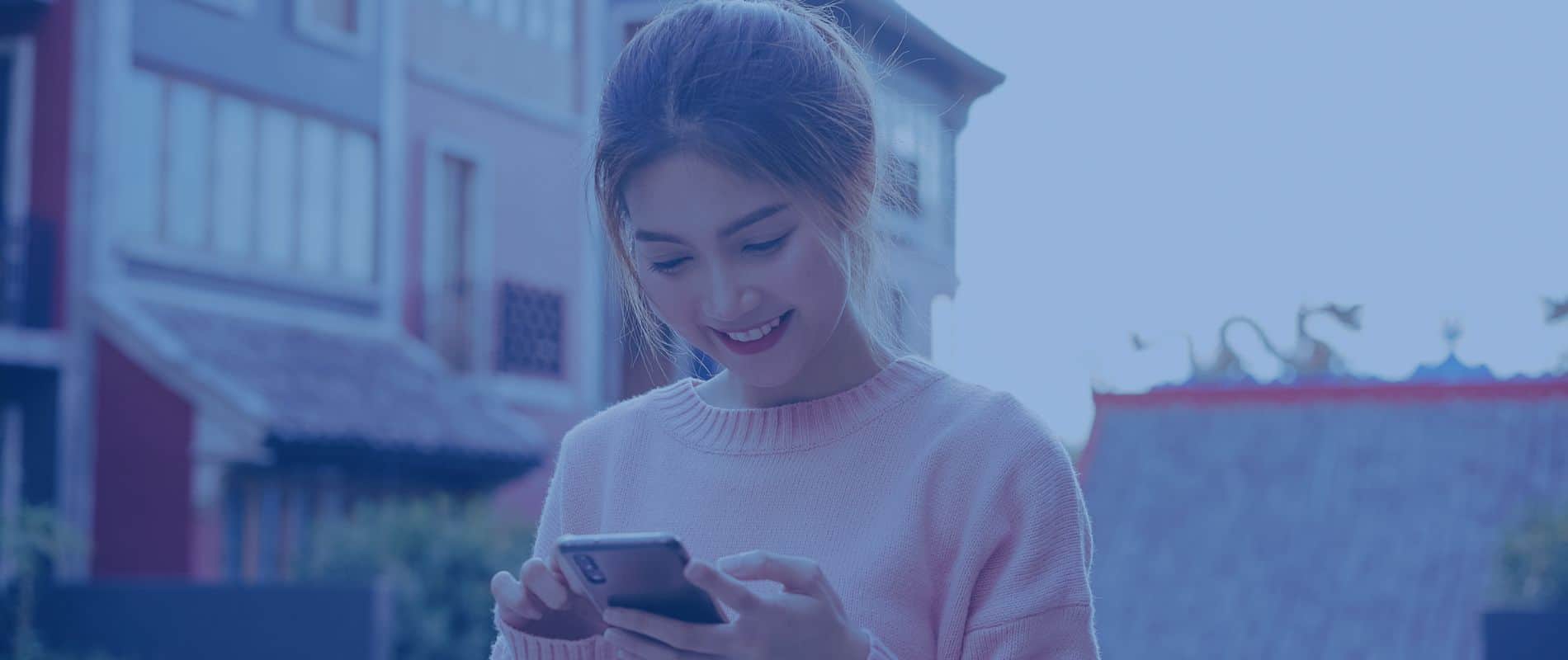
Invest with TFSA
A Tax-Free Savings Account (TFSA) provides you with a flexible way to save for a financial goal, while growing your money tax-free……
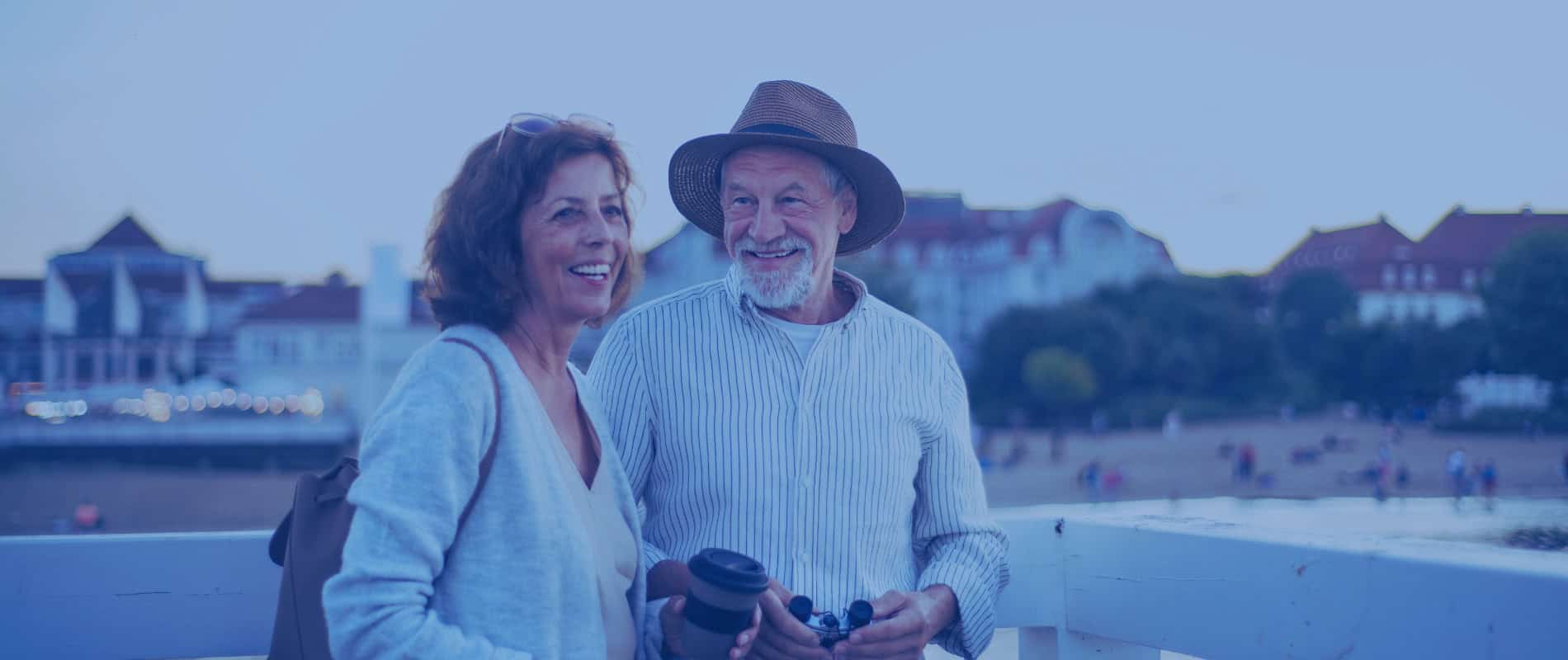
Invest in RRSP-Invest wisely, retire early
According to a recent survey by BMO, due to inflation and rising prices, Canadians now believe they need 1.7 million dollars in savings to retire……